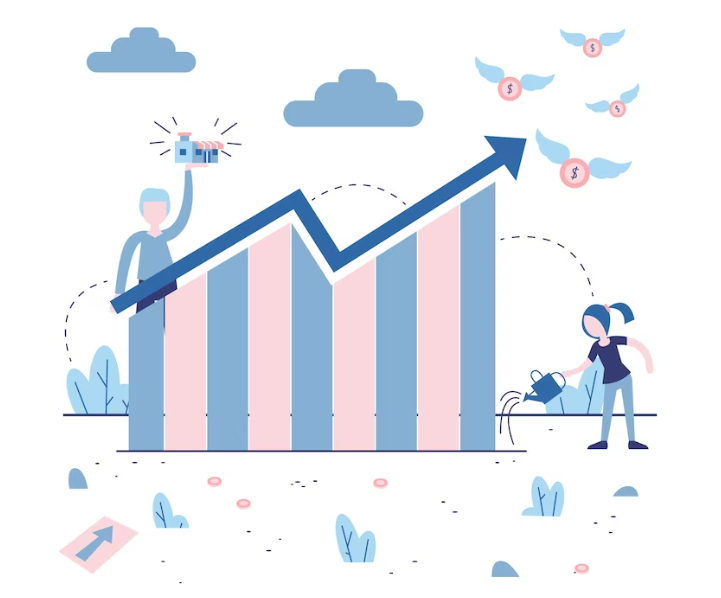
Key Takeaways
- Predictive analytics in eCommerce enables businesses to anticipate customer behavior, optimize marketing strategies, and increase sales.
- Benefits of predictive analytics in eCommerce include enhanced customer segmentation, personalized product recommendations etc.
- Predictive analytics in retail and eCommerce plays a crucial role in demand forecasting, customer lifetime value prediction & many more.
- Implementing predictive analytics in eCommerce marketing automation involves data collection, building predictive models, and integrating with marketing automation platforms.
- Challenges of predictive analytics in eCommerce marketing automation include data privacy compliance, acquiring skills and expertise
- The future of predictive analytics in eCommerce includes enhanced personalization, anticipatory logistics and many more.
Welcome to eCommerce, where businesses strive to provide exceptional customer experiences.
But how do they know what customers want before expressing their needs?
Enter predictive analytics, a game-changing tool in eCommerce marketing automation.
Predictive analytics allows businesses to analyze heaps of data and gain valuable insights.
It helps them make intelligent decisions, create personalized marketing campaigns, and deliver seamless customer experiences.
Join us as we explore the fascinating role of predictive analytics in eCommerce marketing automation and discover how it revolutionizes how businesses engage with their customers.
- What do you mean by predictive analytics in eCommerce?
- Benefits of predictive analytics in eCommerce
- Predictive analytics in retail and eCommerce
- Implementing predictive analytics in eCommerce marketing automation
- Challenges of predictive analytics in eCommerce marketing automation
- Future trends and possibilities
What do you mean by predictive analytics in eCommerce?
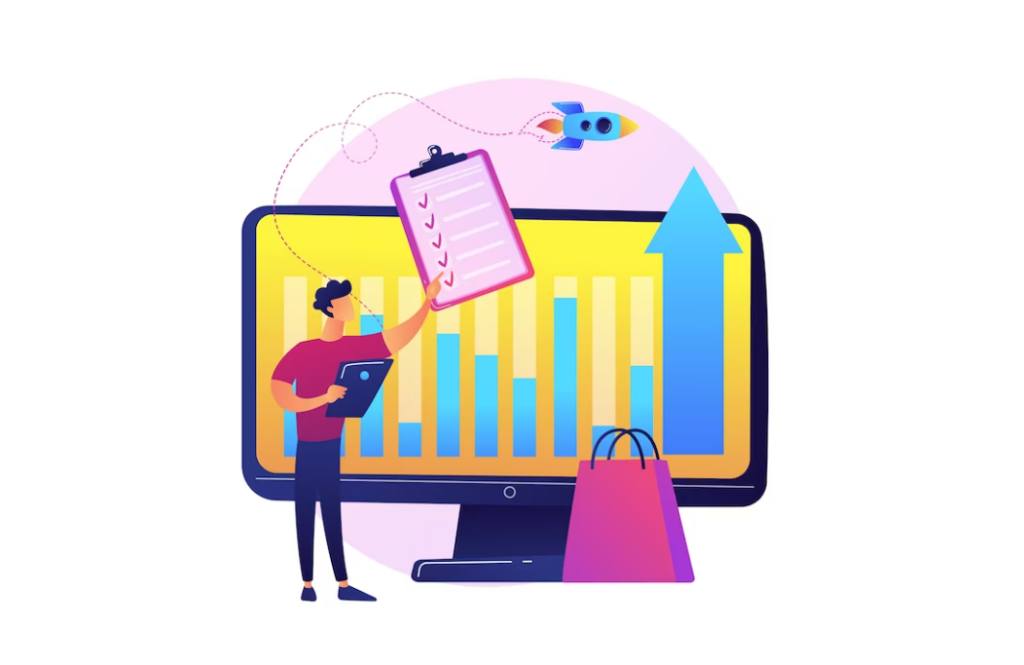
Predictive analytics in eCommerce uses smart data analysis to predict what customers will do next.
It helps online businesses anticipate customer behavior, optimize marketing strategies, and increase sales.
For example, by analyzing a customer’s browsing history and past purchases, predictive analytics can identify those who are likely to make a purchase soon.
With this insight, businesses can target them with personalized offers, improving the chances of converting them into paying customers.
It’s like having a crystal ball that helps businesses understand and meet customer needs, making shopping experiences better and boosting sales.
Benefits of predictive analytics in eCommerce
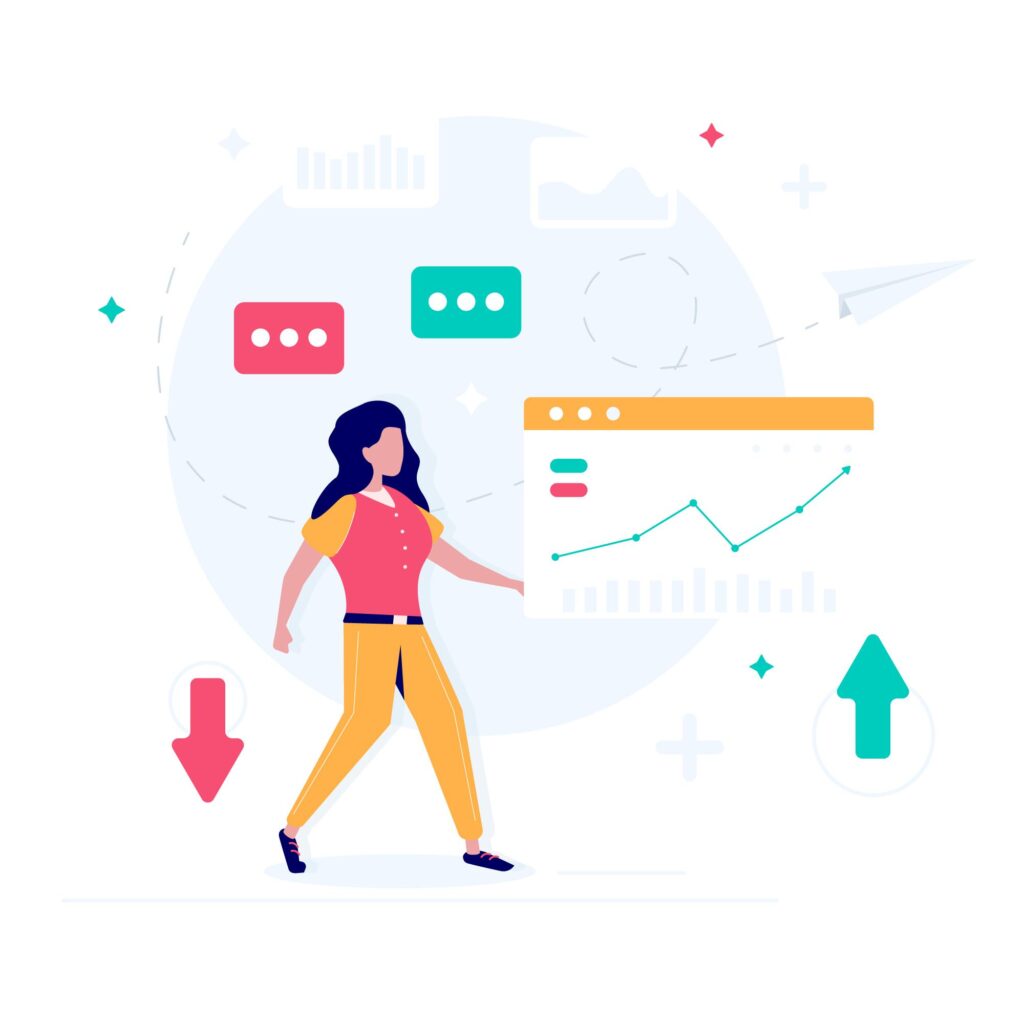
1. Enhanced customer segmentation and targeting
- Predictive analytics allows businesses to create highly precise customer segments based on various data points such as demographics, purchase history, browsing behavior, and more.
- This enables businesses to tailor their marketing messages and offers to specific customer groups, resulting in improved engagement and higher conversion rates.
- For example, a clothing retailer can use predictive analytics to identify a segment of customers who frequently purchase athletic wear and target them with relevant promotions and recommendations.
2. Improved personalized product recommendations
- By analyzing customer behavior and preferences, predictive analytics can generate personalized product recommendations.
- This enhances the customer experience by providing relevant and appealing suggestions based on their individual preferences and browsing history.
- For instance, an online bookstore can use predictive analytics to recommend books to customers based on their previous purchases and browsing patterns, increasing the likelihood of repeat purchases and customer satisfaction.
3. Optimal pricing and promotions strategies
- Predictive analytics enables businesses to analyze pricing patterns, competitor data, and customer response to different price points.
- By understanding customer sensitivity to pricing and demand fluctuations, businesses can optimize their pricing strategies to maximize revenue and profit margins.
- For example, an eCommerce retailer can use predictive analytics to dynamically adjust prices for certain products based on factors like demand, competition, and customer buying patterns, ensuring they remain competitive while maximizing profitability.
4. Anticipating customer churn and retention
- Predictive analytics can identify patterns and indicators of customer churn, allowing businesses to take proactive measures to retain valuable customers.
- By identifying customers who are at risk of churn and implementing targeted retention strategies, such as personalized offers or proactive customer support, businesses can reduce churn rates and improve customer loyalty.
- For instance, a subscription-based service can utilize predictive analytics to identify subscribers who show signs of disengagement and proactively reach out with exclusive offers or content to encourage their continued subscription.
5. Streamlining inventory management and supply chain
- Predictive analytics helps businesses forecast demand, optimize inventory levels, and enhance supply chain efficiency.
- By accurately predicting demand patterns, businesses can avoid stockouts, reduce excess inventory, and ensure timely order fulfillment.
- This leads to improved customer satisfaction by ensuring products are available when customers want them, while also minimizing inventory costs and maximizing operational efficiency.
- Moreover, when integrated with inventory management software, predictive analytics can automate stock adjustments and provide real-time visibility into inventory flow.
6. Enhancing customer lifetime value
- Predictive analytics can estimate the future value of individual customers by analyzing their purchasing behavior, engagement levels, and loyalty.
- This information allows businesses to allocate resources effectively, prioritize high-value customers, and tailor marketing efforts accordingly.
- By focusing on retaining and nurturing valuable customers, businesses can increase customer lifetime value, driving long-term revenue growth.
7. Data-driven decision making
- Predictive analytics provides businesses with data-driven insights and actionable recommendations.
- This empowers decision-makers to make informed choices regarding marketing strategies, inventory management, pricing, and other critical aspects of eCommerce operations.
- By leveraging predictive analytics, businesses can reduce guesswork and make decisions backed by data, leading to more successful outcomes and improved overall performance in the competitive eCommerce landscape.
- Data migration from Amazon RDS to Redshift plays a crucial role in modern data management that supports predictive analytics by enabling seamless data migration and integration, empowering businesses to analyze vast amounts of data efficiently.
Predictive analytics in retail and eCommerce
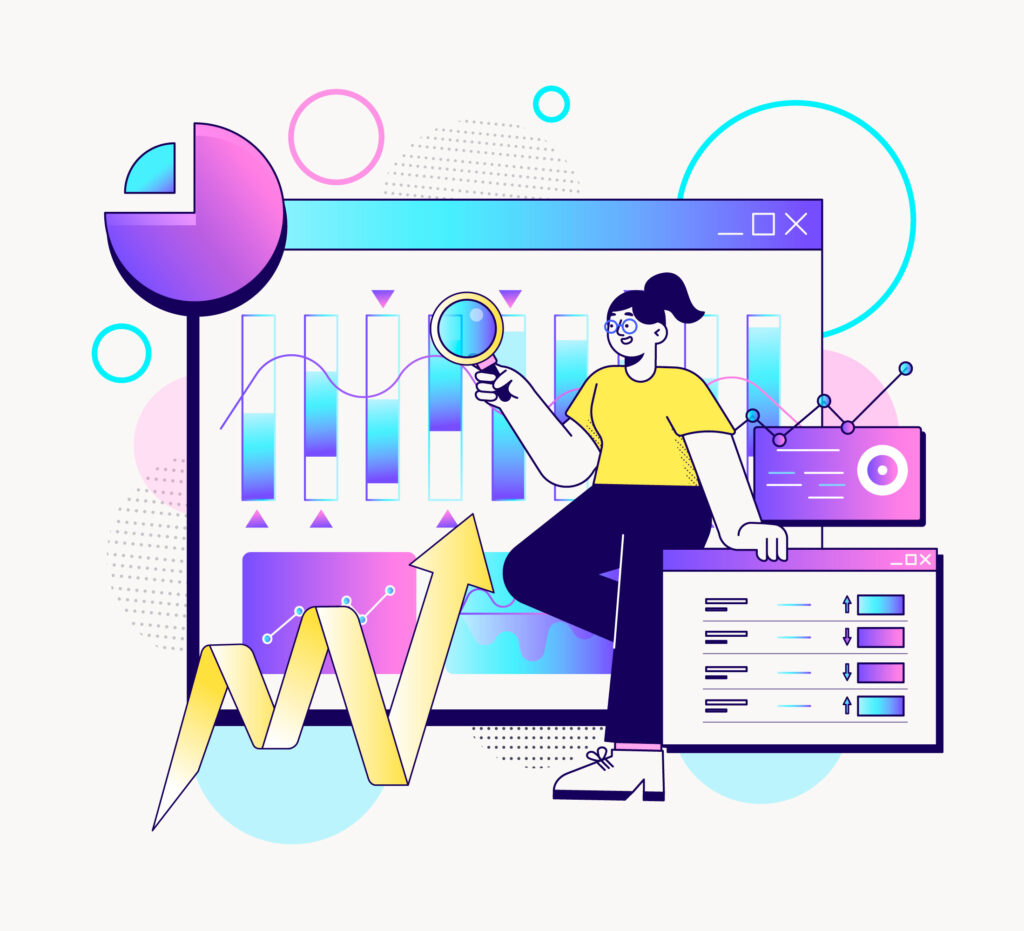
Predictive analytics plays a crucial role in the retail and eCommerce industry, offering a wide range of applications:
1. Demand forecasting and inventory optimization
- By utilizing predictive analytics, retailers can analyze historical sales data, market trends, and external factors like weather patterns and holidays to accurately forecast demand.
- This enables businesses to optimize their inventory levels, ensuring they have the right products available at the right time to meet customer demand.
- By minimizing stockouts and reducing carrying costs associated with excess inventory, retailers can improve customer satisfaction and profitability.
2. Customer lifetime value prediction
- Predictive analytics helps businesses estimate the future value of individual customers by analyzing their purchasing behavior, engagement levels, and loyalty.
- By understanding the potential lifetime value of customers, retailers can allocate resources effectively, prioritize high-value customers, and tailor marketing efforts accordingly.
- This allows for personalized marketing campaigns, loyalty programs, and targeted promotions, ultimately enhancing customer satisfaction and driving long-term revenue growth.
3. Fraud detection and prevention
- Predictive analytics is instrumental in identifying patterns and anomalies in transactional data, helping eCommerce companies detect and prevent fraudulent activities.
- This proactive approach helps retailers minimize financial losses, protect customer information, and maintain a secure online environment, building trust with their customers.
4. Conversion rate optimization
- Predictive analytics enables retailers to analyze customer behavior on their websites, identifying factors that influence conversion rates.
- By understanding which elements of website design, layout, and user experience contribute to higher conversion rates, businesses can optimize their online platforms.
- This can involve implementing personalized recommendations, improving checkout processes, or refining product descriptions, resulting in increased conversions and improved sales performance.
5. Social media analytics and sentiment analysis
Implementing predictive analytics in eCommerce marketing automation
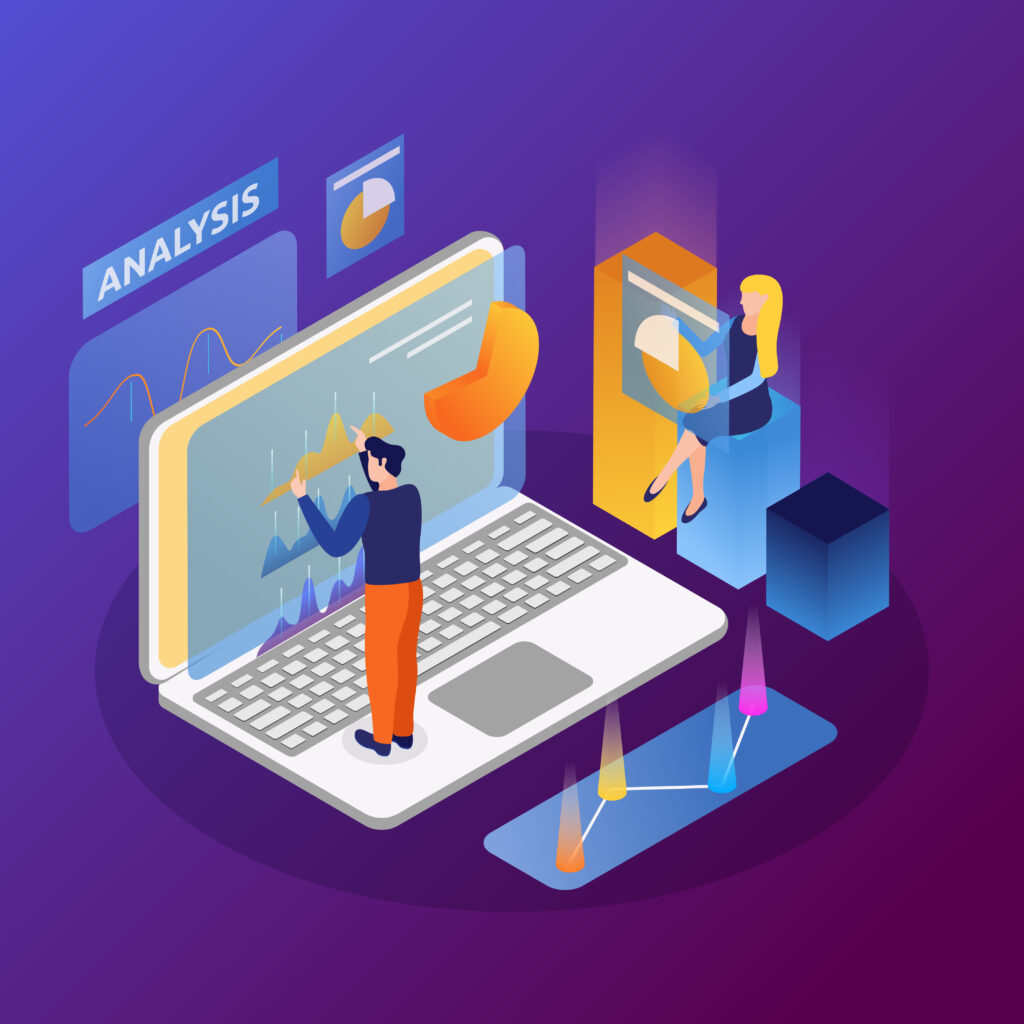
To effectively implement predictive analytics in eCommerce marketing automation, businesses should consider the following steps:
1. Data collection and preprocessing
A. Gathering relevant customer data
- Collect data from various sources, including customer interactions, purchase history, website behavior, and demographic information.
- Ensure the data is comprehensive, accurate, and up-to-date for accurate predictive analysis.
B. Ensuring data quality and accuracy
- Cleanse and preprocess the data to remove duplicates, errors, and inconsistencies.
- Validate and verify the accuracy of the data to ensure reliable predictive modeling.
2. Building predictive models
A. Choosing appropriate algorithms
- Select the right machine learning algorithms based on the specific objectives and nature of the data.
- Common algorithms include regression analysis, decision trees, random forests, and neural networks.
B. Training and validating the models
- Train the predictive models using historical data, allowing them to learn patterns and relationships.
- Validate the models using test data to assess their accuracy and performance.
3. Integration with marketing automation platforms
A. Leveraging customer relationship management systems
- Integrate predictive analytics insights into CRM systems to enrich customer profiles and enhance targeting capabilities.
- Utilize the data to automate personalized email marketing campaigns and customer communications.
B. Syncing predictive insights with email marketing campaigns
- Use predictive analytics to segment customers and tailor email content and offers based on their predicted preferences and behaviors.
- Automate email campaigns to deliver timely, relevant, and personalized messages.
C. Integrating with website personalization tools
- Incorporate predictive analytics into website personalization platforms to dynamically customize the user experience.
- Display targeted product recommendations, personalized content, and promotions based on individual customer preferences.
D. Automating advertising and remarketing efforts
- Utilize predictive analytics to optimize advertising campaigns and allocate budgets effectively.
- Automatically retarget customers with relevant ads based on their browsing and purchasing history.
Challenges of predictive analytics in eCommerce marketing automation
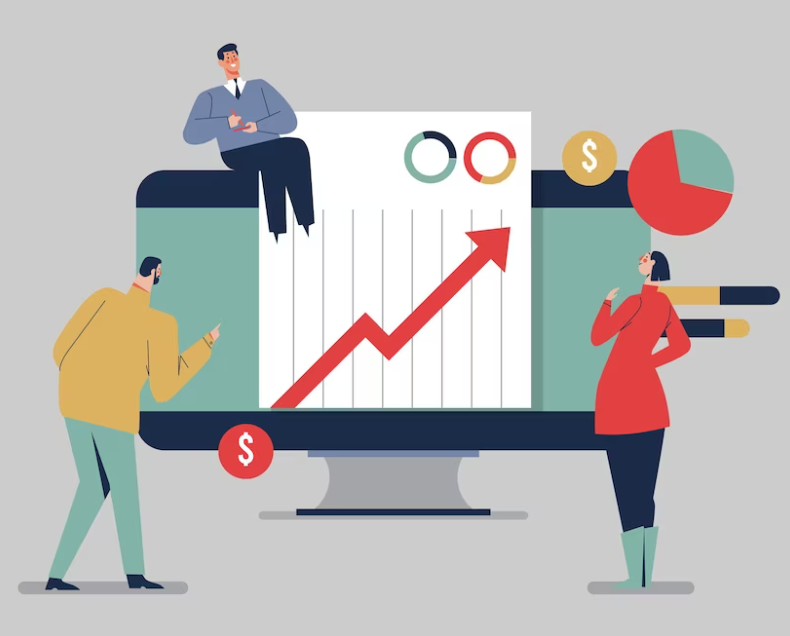
Implementing predictive analytics in eCommerce marketing automation comes with several challenges and considerations, including:
1. Data privacy and compliance
A. Ensuring adherence to data protection regulations
- Businesses must comply with privacy laws and regulations, such as GDPR and CCPA, when collecting and processing customer data.
- Implement appropriate security measures to safeguard customer information.
B. Safeguarding customer information
- Implement robust data encryption, access controls, and monitoring mechanisms.
2. Skills and expertise
A. Hiring or training data scientists
- Businesses may need to hire skilled data scientists or provide training to existing personnel to effectively implement predictive analytics.
- Data scientists can develop and fine-tune predictive models, interpret results, and optimize marketing strategies.
B. Collaborating with analytics professionals
- Work with analytics professionals and domain experts to ensure the accuracy and relevance of predictive analytics models.
- Collaborative efforts can help derive meaningful insights and actionable recommendations.
3. Scalability and infrastructure
A. Handling large volumes of data
- Predictive analytics requires processing and analyzing large datasets, necessitating scalable infrastructure and storage capabilities.
- Consider cloud-based solutions to accommodate increased data volumes and computational requirements.
B. Investing in robust IT infrastructure and cloud services
- Ensure the availability of reliable hardware, software, and networking infrastructure to support predictive analytics processes.
- Leverage cloud services for scalability, flexibility, and cost-effectiveness.
Future trends and possibilities
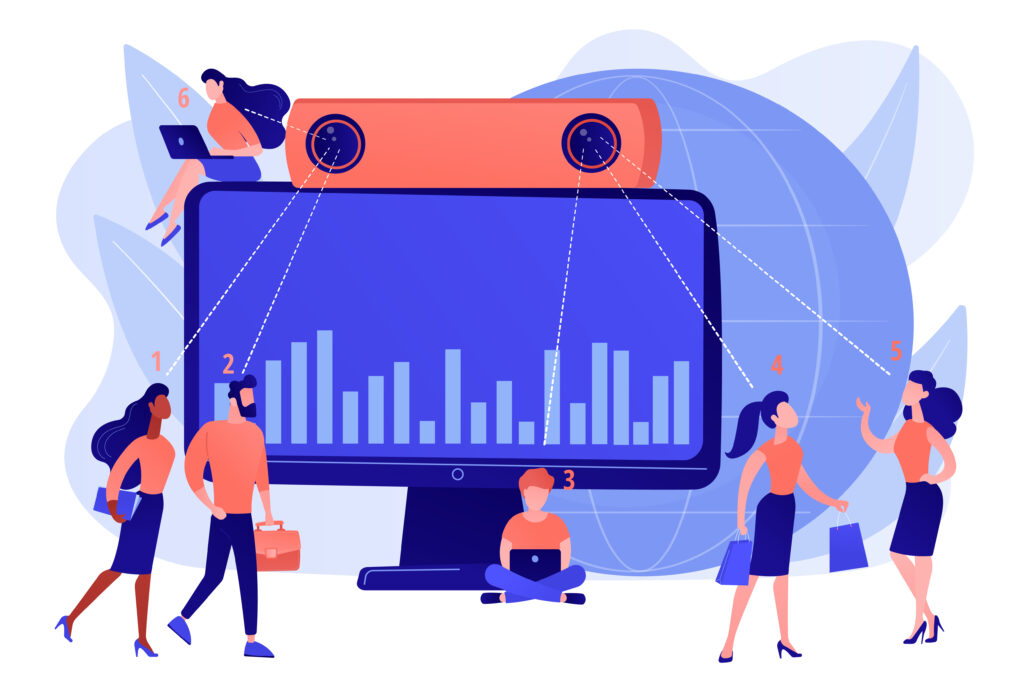
The future of predictive analytics in eCommerce holds immense potential, revolutionizing how businesses operate and engage with customers.
As technology advances and data availability increases, AI in retail and predictive analytics will continue to play a pivotal role in shaping the future of retail and eCommerce.
Here are some key aspects that highlight the exciting prospects:
1. Enhanced personalization and customer experience
- Predictive analytics will enable even more personalized experiences, allowing businesses to anticipate customer needs and provide tailored recommendations.
- Advanced algorithms will leverage vast amounts of customer data to deliver hyper-personalized offers, product suggestions, and customized shopping experiences.
- Imagine a scenario where an online store can accurately predict what a customer wants to buy even before they express their intent, creating seamless and highly satisfying shopping journeys.
2. Anticipatory logistics and supply chain optimization
- Predictive analytics will extend beyond demand forecasting and inventory management to anticipate customer orders before they are placed.
- Retailers will be able to optimize their supply chain, reducing shipping times, and ensuring products are available at the right location and time.
- This anticipatory approach will not only streamline operations but also enhance customer satisfaction by minimizing delivery delays and improving order fulfillment accuracy.
3. AI-powered virtual shopping experiences
- With the integration of artificial intelligence and predictive analytics, virtual shopping experiences will become more immersive and intuitive.
- Leveraging artificial intelligence development services can enhance customer engagement and streamline your eCommerce operations.
- Augmented reality (AR) and virtual reality (VR) technologies will be seamlessly integrated, allowing customers to virtually try on products or visualize how furniture would look in their homes.
- Predictive analytics will play a crucial role in understanding customer preferences, behavior, and purchase patterns, enabling businesses to provide highly relevant and engaging virtual shopping experiences.
4. Proactive customer support and retention
- Predictive analytics will empower businesses to identify customers who are likely to churn or face issues, allowing for proactive customer support and retention strategies.
- By analyzing customer data and behavior, companies can intervene before problems arise, addressing concerns and improving overall customer satisfaction.
- For instance, a subscription-based service can leverage predictive analytics to identify customers showing signs of disengagement, offering personalized incentives or solutions to keep them engaged and loyal.
5. Ethical considerations and data privacy
- As predictive analytics continues to evolve, ensuring ethical use of customer data and maintaining data privacy will be paramount.
- Businesses will need to adopt transparent data practices, obtain proper consent, and prioritize customer privacy to build and maintain trust.
- Regulatory frameworks and industry standards will play a crucial role in shaping the ethical implementation of predictive analytics in eCommerce.
Conclusion
Predictive analytics is like having a crystal ball in the world of eCommerce. It enables businesses to understand their customers better than ever before.
By using predictive analytics, retailers can group customers based on their similarities, offer personalized recommendations, optimize prices and promotions, and ensure they always have the right products in stock.
This not only helps businesses make smarter decisions but also creates targeted marketing campaigns that speak directly to each customer.
As technology continues to advance and more data becomes available, the possibilities for predictive analytics in eCommerce are limitless.
So, buckle up and embrace this powerful tool to stay ahead of the competition, exceed customer expectations, and provide unforgettable shopping experiences in the exciting world of eCommerce.